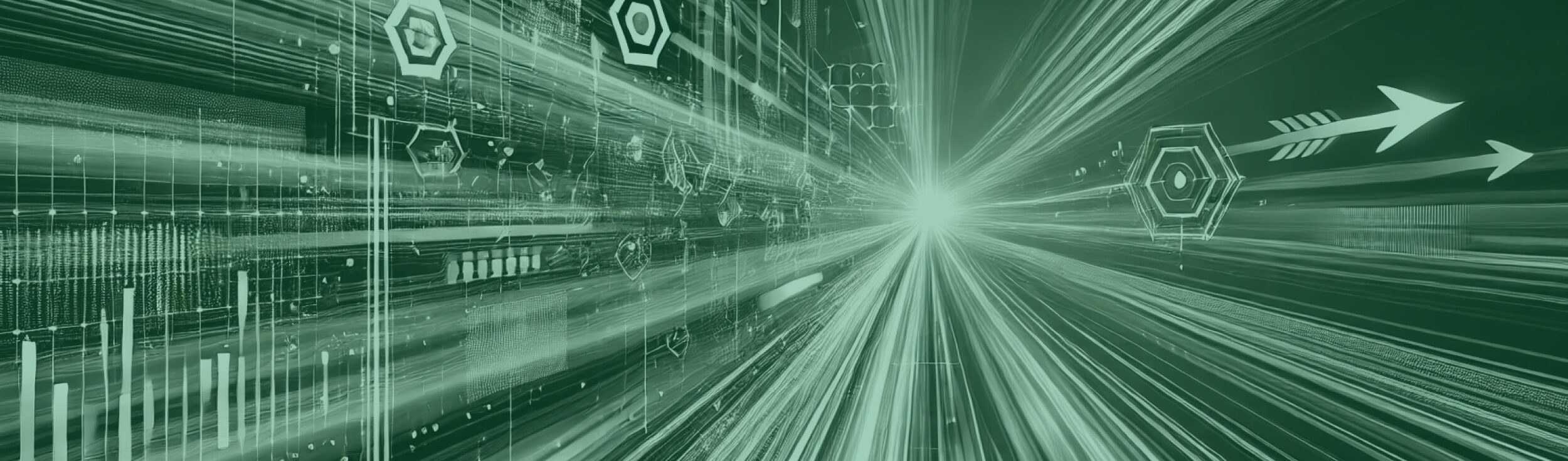
Claude 3.5 Sonnet – the Speed and Direction of Innovation: How Fast is GenAI Progressing?
Anthropic’s recently released Claude 3.5 Sonnet has been making big waves in the GenAI community over the past few weeks. This model marks the most recent blow in the battle among model providers for the crown of most “intelligent” model. Anthropic’s updated Sonnet version boasts improved accuracy rates, a more capable UI, and comparable “intelligence” to the current industry king, GPT 4o. This release has once again reinforced Anthropic’s viability as a notable competitor to OpenAI. Yet more importantly, the release of Sonnet 3.5 is yet another demonstration of the ever-persistent trend of rapid GenAI model growth.
We touched on this topic briefly in our previous post when discussing ways to deal with models being quickly made obsolete by the drastic improvements of their newer counterparts. In mentioning the drastic speed of GenAI model improvement, we prompted the greater question: Exactly how fast are GenAI models actually improving?
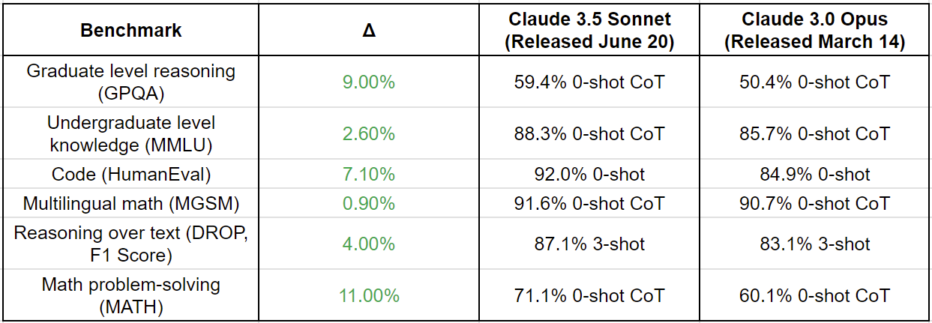
GenAI Growth Rate:
Taking Claude’s benchmarks as an example we can see material improvements, some as high as 11%, on key industry benchmarks in a matter of just three months. These marked improvements demonstrate Sonnet 3.5’s rapid increase in reasoning capabilities, specifically excelling at math problem solving and graduate-level reasoning. Important to note is that Anthropic delivered these significant improvements at a fifth of the price of their previous model, Claude 3 Opus, meaning they improved on all of the typically asymmetric metrics: speed, accuracy, and cost. Yet, this level of iteration has become commonplace and even expected across the highly competitive market of model providers, with similar jumps in model capabilities in the newest Gemini and GPT model suites released in early May.
Benchmarks only tell part of the story, though. The growth rate of model training datasets provides a more quantitative perspective on the rate of model growth over a large timescale and makes identifying trends across a wide range of models easier. These training datasets form the knowledge base on which foundational models are built. Understandably, as these training datasets grow, the capabilities of the models grow in correlation.
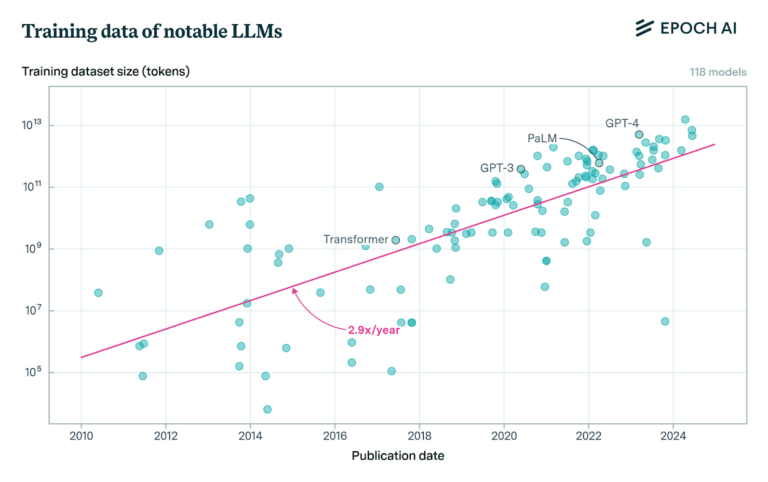
Model training sets have consistently been growing at an exponential rate of 2.9x. To put this growth rate in perspective; Moore’s Law predicts that the number of transistors in a circuit will double approximately every two years, in comparison, over the same two-year period, AI model training datasets would grow by a factor of 8.4x. Since 2020 the average model training data set has grown by 70x. This trend has only become more pronounced since the release of the ubiquitous Transformer model, used in Gemini, Claude and GPT.
This growth rate can be expected to continue into the near future, with estimates of models encountering data scarcity sometime between 2026 and 2032, yet with the promise of processes like synthetic data generation even these dates may end up further delayed.
Where to Next?
With models set to continue growing at this rate, it’s easy to wonder where they may be going next. Intelligence, speed, and cost improvements are obviously all going to be key points of improvement. As OpenAI CEO Sam Altman put it simply, “GPT-5 is just going to be smarter.” Yet even the ‘smartest’ models are still limited by the effectiveness of their application. As a result there’s a growing need for tools that assist in effectively interfacing with these intelligent models to get the most effective outcomes.
This need can be seen being broached by a myriad of small startups but more importantly, can be seen being targeted effectively by the large model providers themselves. In recent model releases we’ve noticed that providers like Anthropic and OpenAI are placing a heavy emphasis on building consumer oriented features to assist in effectively interfacing with their models. In the May GPT 4o release we saw vision and voice functionality added to ChatGPT, with demos showing users able to talk directly to the model while also sharing their in person surroundings. More recently, in the aforementioned Claude 3.5 Sonnet release we saw the introduction of “Artifacts,” a new feature allowing users to generate, view, and edit content like code snippets or graphics in a dedicated workspace alongside their conversations with Claude.
Yet while this heavy emphasis on consumer centric tooling shows great promise for retail users it leaves a void for enterprises who are in a similar state of need for their use cases. We’ve seen massive consulting firms like Accenture capitalizing on this void, leaping to sell their services as the solution. An excerpt from Accenture’s Q3 2024 Earnings Call demonstrating the growth and mass of their GenAI practice:
With over $900 million in new GenAI bookings this quarter, we now have $2 billion in GenAI sales year to date, and we have also achieved $500 million in revenue year to date. This compares to approximately $300 million in sales and roughly $100 million in revenue from GenAI in FY23
Yet, model providers can’t be expected to roll out widely applicable tools to solve this void for all enterprises. In our significant experience integrating GenAI for our enterprise clients, we’ve found that each niche enterprise use case requires its own degree of finesse. And while consulting firms are one solution, even possibly a good one, they’re not the only option.
Effective Enterprise Tools: Fisent as an Example
At Fisent, we solve a very niche yet widespread enterprise issue: effective content ingestion. We apply the capabilities of GenAI within enterprise process automation software, augmenting the pre-existing workflows of a given enterprise to enable better experiences for their clients. Through our service we enable enterprises to have greater accuracy, auditability and speed in the processing and ingestion of content. By applying GenAI in this tailored manner, we are able to provide highly capable solutions that are personalized to our clients, unlike umbrella solutions that may eventually be rolled out by foundational model providers. Additionally, Fisent’s breadth of experience addressing this specific issue means that we have pre-built tools, processes, and endpoints that allow us to efficiently, and cost effectively integrate within any enterprise, unlike the more costly and time-intensive solutions offered by the large consulting firms.
Regardless of the method of application, it’s indisputable that GenAI is progressing rapidly. While retail users are benefiting from software that makes applying GenAI easier than ever, it’s clear that enterprises can greatly benefit from more effective tooling. If you’re interested in learning more about Fisent’s GenAI Solution, consider reading our BizAI page. Alternatively, if you’re interested in learning how to evaluate the wide range of available LLMs, check out our previous post.