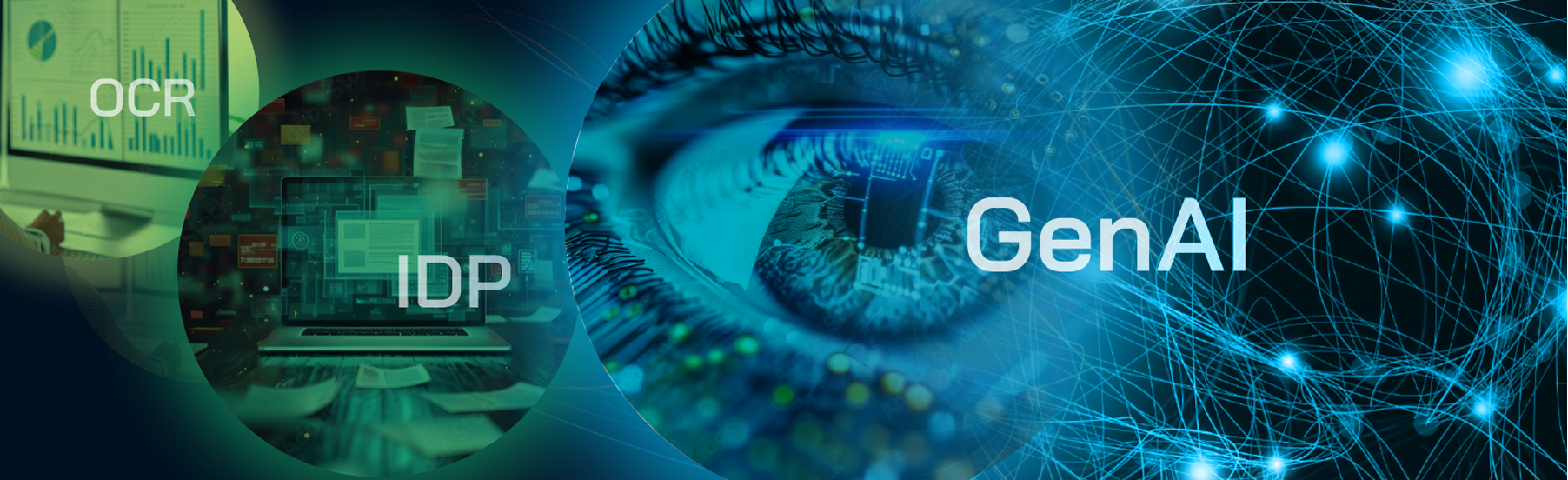
The Evolution of Digital Content Processing: OCR, IDP, GenAI
Businesses handle an immense amount of content daily, with most mission-critical processes being traced back to ingested content. Whether that be processing purchase orders to ship products on time or accurately interpreting refund requests to guarantee a positive customer experience.
While technologies like Optical Character Recognition (OCR) and Intelligent Document Processing (IDP) have helped accelerate content processing, the reality of non-standard and unstructured content has historically prevented enterprises from fully automating their processes from end-to-end. Yet today, with Applied GenAI Process Automation tools this is no longer the case. Many of IDP and OCR’s technical limitations have been bridged with the capabilities enabled through the strategic application of GenAI models.
In this article, we will clearly define the capabilities and limitations of OCR and IDP, and outline the key technical differences between these technologies and new GenAI-powered content processing solutions like our own Fisent BizAI.
Available Content Processing Technology: Definition, Capabilities and Limitations
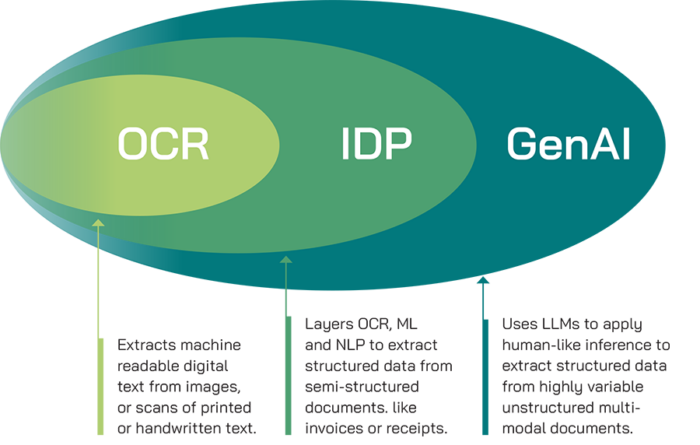
Optical Character Recognition (OCR):
OCR is one of the most fundamental document processing technologies, in fact, it lies at the heart of almost every modern content processing pipeline. This technology transforms scanned copies or images of text and handwriting into machine readable text, that can then be manipulated or extracted for use within a software solution.
Business workflow with structured documents, where content appears in a fixed format with data points in predefined locations can be processed by OCR systems. Using a defined set of rules and document coordinates, these OCR systems will extract data from specified fields of a given document. Think, pulling the TINs from tax forms or DOBs from passports.
OCR limitations:
- Limited Sophistication – OCR does not contain any “inference capabilities”, meaning it does not understand the relationships between the content it is extracting, so it can only automate the processing of structured documents where inputs follow a consistent format.
- Lacks Adaptability – Due to OCR requiring predefined layouts and schemas for document processing, any changes made to document formats or labels, in turn, requires retraining of the models used within OCR solutions, which can become a significant time investment.
- Limited applicability – while OCR is likely the most widely-used document processing technology, it has a limited set of applicability due to the rigidity of the technology.
Intelligent Document Processing:
IDP builds on the capabilities of OCR enabling enterprises to automate the processing of semi-structured documents, where the content format varies but still follows a rough pattern (ie; Invoices or Purchase Orders). IDP does this by applying probabilistic machine learning models that learn from the data they process and by applying natural language processing (NLP) techniques to ‘intelligently’ gather data from documents with slightly varying formats.
IDP solutions train on processed content, with accuracy gradually improving through learning from human corrections (“reinforcement”). This means IDP solutions require human intervention, specifically for documents that vary from their training set. This method of progressive improvement means that IDP solutions are template based and typically require separate models for various document types within a business process. For example, a company looking to automate its purchase order processing would require separate IDP models to process invoices, purchase orders, receipts, and shipping notes.
IDP Limitations:
- Total Cost of Ownership – IDP requires training on processed content and regular human validation for outliers/complex cases
- Limited Learning – Struggles to adapt dynamically to new types of documents or content without training models.
- Limited Result Explainability – IDP models are complex making it incredibly difficult for a non-expert to understand how the probabilistic model achieved its results.
Applied GenAI Process Automation:
Finally, Applied GenAI Process Automation tools provide an option for processing content of all types, excelling at handling unstructured content. Unstructured content follows no format guidelines, is highly varied and possibly even multimodal. Some examples of unstructured content include contracts, emails with attachments or even complex legal documents.
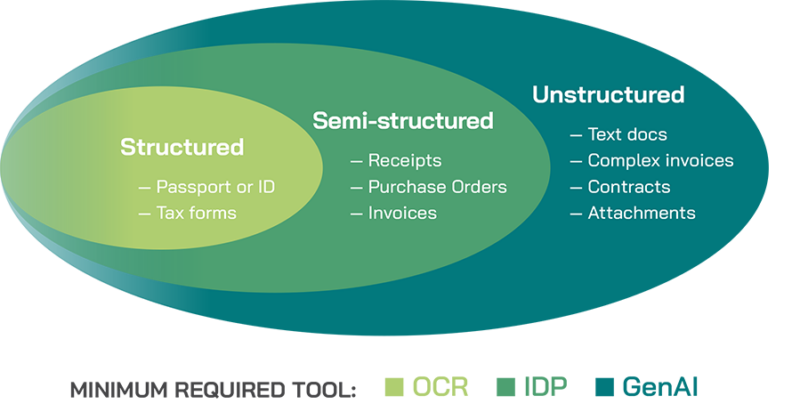
Similar to IDP, these GenAI tools apply OCR to digitize text. However, instead of layering NLP or vertically trained ML models to identify key data fields and entities, they strategically apply LLMs to gather context, derive semantic meaning, and intelligently extract data from content, regardless of format or language. Furthermore, with multi-modal support for text, audio, video and images these models go beyond the scope of OCR and IDP by allowing content analysis beyond just text based documents.
These capabilities allow for some unique use cases typically out of scope for an IDP or OCR solution. Some examples include:
- Process PO received as an audio file in the same pipeline as POs received attached to an email or even embedded within an Excel sheet.
- Extract and structure critical data from a complex legal document such as listing the ‘authorities’ provided within a Power of Attorney.
Business Impacts of GenAI-Powered Content Processing:
Increased Accuracy and Efficiency
With strong contextual understanding, GenAI Process Automation tools excel at accurately extracting data. At Fisent, our BizAI solution is leading the industry in Applied GenAI Process Automation. In most of our use cases we’ve observed accuracy rates exceeding 93%, even with complex, multi-modal documents. Furthermore, by bridging the automation gap and enabling automated content processing of unstructured documents, organizations can fully automate key business processes from end-to-end. This means drastically reduced processing time and more time made available for employees to complete higher value tasks. For example, PC Connection recently reduced their Purchase Order processing time by 98% as a result of integrating BizAI into their workflow.
Scalability, Security and Adaptability
Progressing from IDP to GenAI powered solutions doesn’t just provide users with the ability to process unstructured content, but also means continuous human oversight and access to your data for model training is no longer required. A tool like Fisent’s BizAI retains no data instead applying prompt engineering and state of the art LLMs without training on processed content. This pattern has a couple of positive implications: Content processing technologies have significantly evolved over time, with OCR and IDP providing foundational automation for structured and semi-structured documents. However, they fall short in fully automating processes involving unstructured or highly varied content. Applied GenAI Process Automation solutions, on the other hand, overcome these limitations by leveraging advanced models capable of interpreting and extracting the meaning from diverse content types, whether text, audio, or images. By adopting solutions like Fisent’s BizAI, businesses can not only boost efficiency and accuracy but also scale with future-proof technologies, ensuring continued process improvements and faster turnaround times.
Conclusion: